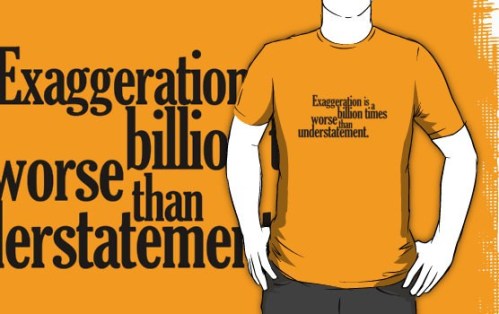
There is a new paper
Linda O. Mearns, Ray Arritt, Sébastien Biner, Melissa S. Bukovsky, Seth McGinnis, Stephan Sain, Daniel Caya, James Correia, Jr., Dave Flory, William Gutowski, Eugene S. Takle, Richard Jones, Ruby Leung, Wilfran Moufouma-Okia, Larry McDaniel, Ana M. B. Nunes, Yun Qian, John Roads, Lisa Sloan, Mark Snyder, 2012: The North American Regional Climate Change Assessment Program: Overview of Phase I Results. Bull. Amer.Met Soc. September issue. pp 1337-1362.
that provides further documentation of the level of skill of dynamic downscaling. It is a very important new contribution which will be widely cited. The participants in the North American Regional Climate Change Assessment Program are listed here.
However, it significantly overstates the significance of its findings in terms of its application to the multi-decadal prediction of regional climate.
The paper is even highlighted on the cover of the September 2012 issue of BAMS, with the caption for the cover in the Table of Contents that reads
“Regional models are the foundation of research and services as planning for climate change requires more specific information than can be provided by global models. The North American Regional Climate Change Assessment Programs (Mearns et al., page 1337) evaluates uncertainties in using such models….”
Actually, as outlined below, the Mearns et al 2012 paper, while providing valuable new insight into one type of regional dynamic downscaling, is misrepresenting what these models can skillfully provide with respect to “climate change”.
The study uses observational data (from a Reanalysis) to drive the regional models. Using the classification we have introduced in our papers (see below), this is a type 2 dynamic downscaling study.
The Mearns et al 2012 paper only provides an upper bound of what is possible with respect to their goal to provide
“uncertainties in regional scale projections of future climate and produce high resolution climate change scenarios using multiple regional climate models (RCMs) nested within atmosphere ocean general circulation models (AOGCMs) forced with the A2 SRES scenario.”
The type of downscaling used in a study is a critically important point that needs to be emphasized when dynamic downscaling studies are presented. Indeed, the new paper seeks to just replicate the current climate, NOT changes in climate statistics over the time period of the model runs.
It is even more challenging to skillfully predict CHANGES in regional climate which is what is required if the RCMs are to add any value for predicting climate in the coming decades.
The abstract and their short capsule reads [highlight added]
The North American Regional Climate Change Assessment Program is an international effort designed to investigate the uncertainties in regional scale projections of future climate and produce high resolution climate change scenarios using multiple regional climate models (RCMs) nested within atmosphere ocean general circulation models (AOGCMs) forced with the A2 SRES scenario, with a common domain covering the conterminous US, northern Mexico, and most of Canada. The program also includes an evaluation component (Phase I) wherein the participating RCMs, with a grid spacing 50 km, are nested within 25 years of NCEP/DOE global reanalysis II.
We provide an overview of our evaluations of the Phase I domain-wide simulations focusing on monthly and seasonal temperature and precipitation, as well as more detailed investigation of four sub-regions. We determine the overall quality of the simulations, comparing the model performances with each other as well as with other regional model evaluations over North America. The metrics we use do differentiate among the models, but, as found in previous studies, it is not possible to determine a ‘best’ model among them. The ensemble average of the six models does not perform best for all measures, as has been reported in a number of global climate model studies. The subset ensemble of the 2 models using spectral nudging is more often successful for domain wide root mean square error (RMSE), especially for temperature. This evaluation phase of NARCCAP will inform later program elements concerning differentially weighting the models for use in producing robust regional probabilities of future climate change.
Capsule
This article presents overview results and comparisons with observations for temperature and precipitation from the six regional climate models used in NARCCAP driven by NCEP/DOE Reanalysis II (R2) boundary conditions for 1980 through 2004.
Using the types of dynamic downscaling that we present in the articles
Castro, C.L., R.A. Pielke Sr., and G. Leoncini, 2005: Dynamical downscaling: Assessment of value retained and added using the Regional Atmospheric Modeling System (RAMS). J. Geophys. Res. – Atmospheres, 110, No. D5, D05108, doi:10.1029/2004JD004721.
Pielke Sr., R.A., and R.L. Wilby, 2012: Regional climate downscaling – what’s the point? Eos Forum, 93, No. 5, 52-53, doi:10.1029/2012EO050008.
the Mearns et al 2012 paper is a Type 2 downscaling. It provides an upper bound on the skill possible from Type 3 and Type 4 downscaling, since real world observations are used to constrain the model simulations (through the lateral boundary conditions, and from interior nudging if used).
These types of downscaling are defined in the Castro et al 2005 and Pielke and Wilby 2012 papers as
Type 1 downscaling is used for short-term, numerical weather prediction. In dynamic type 1 downscaling the regional model includes initial conditions from observations. In type 1 statistical downscaling the regression relationships are developed from observed data and the type 1 dynamic model predictions.
Type 2 dynamic downscaling refers to regional weather (or climate) simulations [e.g., Feser et al., 2011] in which the regional model’s initial atmospheric conditions are forgotten (i.e., the predictions do not depend on the specific initial conditions) but results still depend on the lateral boundary conditions from a global numerical weather prediction where initial observed atmospheric conditions are not yet forgotten or are from a global reanalysis. Type 2 statistical downscaling uses the regression relationships developed for type 1 statistical downscaling except that the input variables are from the type 2 weather (or climate) simulation. Downscaling from reanalysis products (type 2 downscaling) defines the maximum forecast skill that is achievable with type 3 and type 4 downscaling.
Type 3 dynamic downscaling takes lateral boundary conditions from a global model prediction forced by specified real world surface boundary conditions such as seasonal weather predictions based on observed sea surface temperatures, but the initial observed atmospheric conditions in the global model are forgotten [e.g., Castro et al., 2007]. Type 3 statistical downscaling uses the regression relationships developed for type 1 statistical downscaling except using the variables from the global model prediction forced by specified real-world surface boundary conditions.
Type 4 dynamic downscaling takes lateral boundary conditions from an Earth system model in which coupled interactions among the atmosphere, ocean, biosphere, and cryosphere are predicted [e.g., Solomon et al.,
2007]. Other than terrain, all other components of the climate system are calculated by the model except for human forcings, including greenhouse gas emissions scenarios, which are prescribed. Type 4 dynamic
downscaling is widely used to provide policy makers with impacts from climate decades into the future. Type 4 statistical downscaling uses transfer functions developed for the present climate, fed with large scale atmospheric information taken from Earth system models representing future climate conditions. It is assumed that statistical relationships between real-world surface observations and large-scale weather patterns will not change. Type 4 downscaling has practical value but with the very important caveat that it should be used for model sensitivity experiments and not as predictions [e.g., Pielke, 2002; Prudhomme et al., 2010].
Because real-world observational constraints diminish from type 1 to type 4 downscaling, uncertainty grows as more climate variables must be predicted by models, rather than obtained from observations.
The Mearns et al 2012 study concludes with the claim that
Our goal was to provide an overview of the relative performances of the six models both individually and as an ensemble with regard to temperature and precipitation. We have shown that all the models can simulate aspects of climate well, implying that they all can provide useful information about climate change. In particular, the results from phase I of NARCCAP will be used to establish uncertainty due to boundary conditions as well as final weighting of the models for the development of regional probabilities of climate change.
First, as documented in the article, the difference between the models and the observations are actually significant. To claim that
“all the models can simulate aspects of climate well”
is not a robust claim. What is meant by “well”? The tables and figures in the article document significant biases in the temperatures and precipitation even for the current climate type 2 downscaling simulations.
Even more significantly, their type 2 downscaling study does NOT imply
“that they all can provide useful information about climate change”!
The Mearns et al 2012 study did not look at the issue of their skill to predict CHANGES in climate statistics. For this they must examine type 4 downscaling skill, which they did not do.
In the context of the skill achieved with type 2 dynamic downscaling, this is an important, useful study. However, to use the results of this type 2 downscaling study by Mearns et al 2012 to provide
“….final weighting of the models for the development of regional probabilities of climate change”
is a gross overstatement of what they accomplished. One cannot use type 2 downscaling to make claims about the accuracy of type 4 downscaling.
I am e-mailing the authors of the Mearns et al 2012 paper to request their response to my comments. Each of them are well-respected colleagues and I will post their replies when they respond.
source of image